In the world of business, demand forecasting is one of those essential but challenging tasks. Every company wants to predict future sales and customer demand as accurately as possible. A reliable forecast allows businesses to plan production, manage inventory, and allocate resources efficiently.
However, achieving 100% demand forecast accuracy is nearly impossible. In reality, even the most advanced forecasting models come with a margin of error, leaving businesses to cope with the unavoidable impacts of inaccuracy.
In this post, we’ll explore why perfect accuracy is unattainable, the realistic targets companies can aim for, the potential impacts of forecast errors, and practical strategies for managing these risks.
Before we go further into this topic, don’t forget to follow my LinkedIn account. You’ll get more helpful insights on supply chain management there.
Table of Contents
Why 100% Demand Forecast Accuracy is Unrealistic
Imagine being able to predict every shift in customer behavior, every market fluctuation, and every external factor that could impact demand. This level of precision is an ideal scenario, but one that’s far from reality.
In demand forecasting, numerous variables are at play, and many of them are inherently unpredictable. While we can use historical data, market trends, and economic indicators to make educated guesses, these factors can change unexpectedly.
Demand is influenced by external events like economic shifts, political changes, and natural disasters, as well as internal factors like marketing campaigns or product launches. These influences are often volatile, making it difficult to capture every possible outcome. Even the best forecasting models have limitations. They rely heavily on historical data, which may not always be relevant in a rapidly changing environment. For example, models built on pre-pandemic sales data were likely skewed by the sudden changes brought by COVID-19.
As a result, no matter how refined or sophisticated the forecasting approach, demand prediction will always involve a degree of uncertainty. Companies can certainly improve their accuracy, but aiming for 100% perfection isn’t realistic. Instead, the goal should be to minimize the error rate as much as possible and prepare for the inevitable discrepancies.
Realistic Forecast Accuracy Targets Across Industries
Given the complexity and variability involved, companies in different industries have developed realistic forecast accuracy targets. Generally, aiming for a forecast accuracy of 70% to 90% is considered strong and achievable. For most consumer goods and retail companies, a target of around 80-90% on short-term forecasts is seen as a solid benchmark. These sectors often face high demand variability, which makes perfect accuracy nearly unattainable but still allows for a relatively high level of precision due to consistent data on buying patterns and seasonal trends.
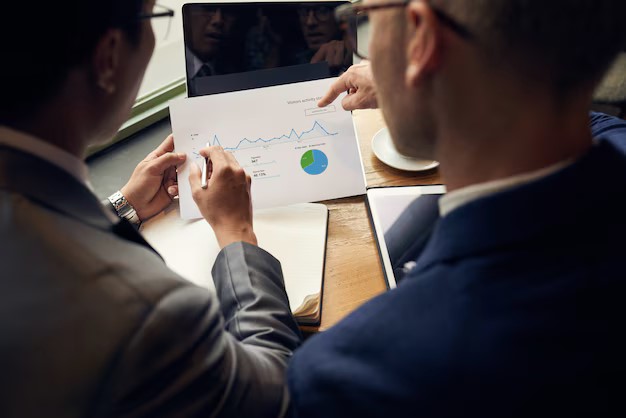
In manufacturing and B2B environments, an 85% or higher accuracy level is a practical goal, especially for stable, long-term contracts or products with consistent demand. Companies with more predictable customer needs or long-term agreements can leverage this stability to achieve higher accuracy.
However, in industries like fashion or seasonal products, the demand can be more erratic, so accuracy targets may be closer to 70-80%. These industries see rapid changes in customer preferences and market trends, leading to more frequent inaccuracies. Ultimately, the goal of demand forecasting isn’t to achieve perfect accuracy but to continuously reduce error rates and adapt forecasts based on real-time information and trends.
Instead of focusing solely on achieving a specific accuracy percentage, companies often prioritize reducing forecast errors. Metrics like Mean Absolute Percentage Error (MAPE) help track forecasting accuracy more meaningfully, ensuring that efforts are geared towards continuous improvement rather than the unattainable goal of 100% precision.
You might also like:
- Without the Right KPIs, You Can’t See the True Performance of Your Supply Chain
- How to Fix Major Supply Chain Management Mistakes Without Hurting Team Morale
The Impact of Forecast Inaccuracy on Business
Even with a forecast accuracy target of 70-90%, the remaining 10-30% margin of error can have significant consequences. Forecast inaccuracies affect several aspects of a business, from inventory management to customer satisfaction and profitability.
The most common and immediate impact is on inventory levels. When demand is overestimated, businesses may end up with excess inventory, which ties up capital and increases storage costs. In sectors with perishable goods or short product lifecycles, excess stock can lead to wastage or the need for heavy discounting, eroding profitability.
On the flip side, underestimating demand results in stockouts, causing delays or missed sales opportunities. Customers may turn to competitors when they can’t find what they need, leading to lost revenue and potentially damaging brand loyalty. This situation is especially critical in highly competitive markets, where customer expectations for availability and quick service are high.
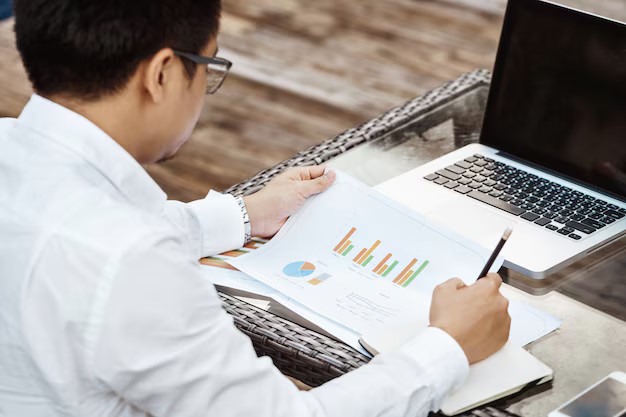
Operational inefficiencies are another impact of forecast inaccuracies. When forecasts are off, production schedules may need last-minute adjustments, leading to disruptions and higher costs. For instance, unexpected demand surges may require overtime labor or rush orders from suppliers, both of which increase operational expenses. Conversely, overestimating demand might result in underutilized resources, driving up per-unit production costs.
Logistics is also affected by forecast inaccuracies. Overestimated demand can lead to underfilled shipments, raising shipping costs per unit, while underestimated demand might lead to rushed shipping, which is often more expensive. All these logistics fluctuations further strain profitability and complicate supply chain management.
Customer satisfaction takes a hit when inaccurate forecasts lead to stockouts or delays. Modern customers expect reliability and consistency, and they’re more likely to switch brands if a company can’t meet their needs consistently. Dissatisfied customers might not only leave but also share their negative experiences with others, potentially damaging the brand’s reputation.
Financial impacts from forecast errors are significant as well. Stockouts result in missed sales opportunities, reducing potential revenue, while overstock can lead to markdowns and profit losses. When inventory sits idle, it ties up capital that could have been invested elsewhere, creating cash flow strains that limit the company’s financial flexibility.
You might also like:
- The Hidden Risks of System Automation in Supply Chain Management
- Port Congestion Crisis: How to Keep Your Supply Chain Moving Smoothly
Strategies to Mitigate Forecast Inaccuracies
Given the impacts of forecast inaccuracy, businesses need to focus on strategies that can reduce the risks and help them manage demand fluctuations effectively.
One of the most common approaches is maintaining safety stock, or buffer inventory. While holding additional inventory can increase carrying costs, it provides a cushion against unexpected demand spikes, reducing the likelihood of stockouts and lost sales. Companies need to balance the size of their safety stock with carrying costs, using demand data and historical patterns to set optimal levels.
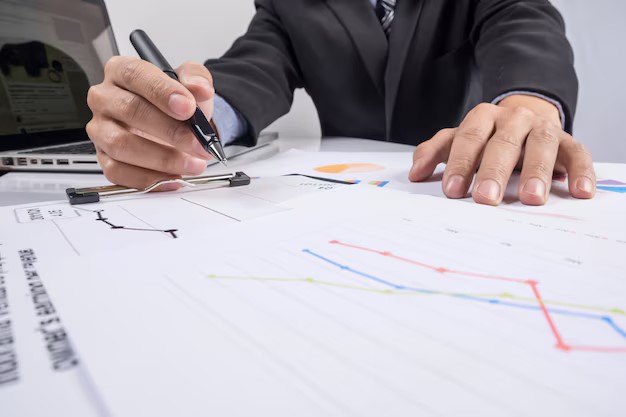
Flexible production and supply chains are another effective way to mitigate the risks associated with forecast inaccuracy. Agility in production allows businesses to scale operations up or down quickly in response to demand changes, minimizing waste and preventing shortages. Multi-supplier sourcing, where companies work with several suppliers rather than relying on a single source, can also add flexibility and reduce the risk of bottlenecks.
Another strategy that’s increasingly popular is demand-driven replenishment. This approach shifts the focus from forecasted demand to actual demand data. By using real-time sales data or customer orders to inform production and inventory decisions, companies can reduce their reliance on forecasts alone. Demand-driven replenishment allows businesses to adapt to real demand changes, improving their responsiveness and reducing the chances of overstock or stockouts.
Collaborative forecasting is another valuable strategy. Involving key stakeholders across functions—such as sales, marketing, and supply chain—helps improve forecast accuracy. By incorporating insights from different departments, businesses gain a more comprehensive view of demand drivers. Similarly, collaboration with suppliers can lead to better alignment on production and replenishment schedules, reducing the risk of discrepancies between forecasted and actual demand.
Advanced analytics and machine learning are increasingly being used to enhance forecasting accuracy. Predictive analytics can identify patterns in historical data, helping companies refine their forecasts continuously. Machine learning models can adapt to new information over time, providing updated forecasts as market conditions change. With the ability to analyze vast amounts of data quickly, these tools allow businesses to improve their forecast reliability and respond more effectively to demand fluctuations.
Conclusion
In demand forecasting, perfection is an unrealistic goal. Despite all efforts, demand predictions will always involve some degree of error, especially in volatile markets. Rather than striving for 100% accuracy, businesses should focus on setting realistic targets, continuously refining their forecasts, and building resilience into their operations. By aiming for a balance between forecast accuracy and flexibility, companies can better prepare for the unexpected and reduce the negative impacts of forecast errors.
Demand forecasting is a journey of continuous improvement, not a destination. By implementing strategies like safety stock, flexible production, demand-driven replenishment, collaborative forecasting, and advanced analytics, businesses can mitigate the risks of forecast inaccuracy. Embracing these approaches not only improves resilience but also builds a foundation for sustainable growth, even in the face of uncertainty.
The goal is to stay responsive and adaptable, using forecasts as a guide while maintaining the flexibility to navigate the inevitable changes in customer demand. In this way, companies can turn forecast challenges into opportunities for innovation and stronger customer relationships.
I hope you find it helpful!
Please share this article with your colleagues so they can also benefit. For more insights on supply chain management, follow my LinkedIn account. You’re free to use all articles on this blog for any purpose, even for commercial use, without needing to give credit.